The tinyML Summit 2020 was by no means tiny. With almost 400 attendees, the conference saw a 150% increase in attendance compared to the previous year. This led to a last-minute change in venue to accommodate the significant growth.
We were delighted to be Gold Sponsor for the event, showcasing XMOS and revealing our new crossover processor for the AIoT, xcore®.ai. Our poster presentation shared the xcore.ai architecture for the first time and received extremely positive feedback, particularly around our power and latency benchmarks. This was supported by a timely, exclusive press announcement in EETimes.
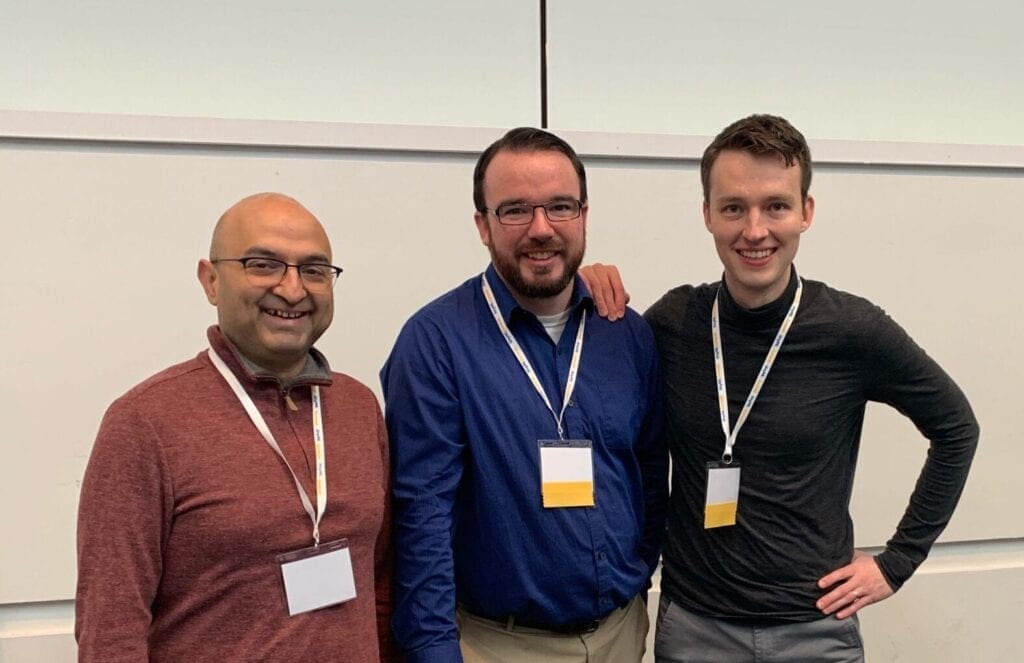
Over the course of the two days, there was a wide selection of talks, panel discussions and poster presentations, all exploring the challenges and solutions of tinyML.
The first sessions on Wednesday morning focused on the latest techniques for designing, compressing, and training neural networks specifically with tiny devices in mind – crucial for more complex device/sensor level AI. A panel discussion followed, exploring how to build a tinyML company. Led by Chris Rowen, co-founder and CEO of BabbleLabs, the panel included representatives from Clear Ventures, MicroTech Ventures, Sorenson Ventures and Qualcomm. They determined that in the face of a wide and fast-moving industry, companies need to decide whether they are delivering either technology or products and avoid tackling both. The afternoon session focused on tinyML systems and applications. Kris Pister from UC Berkeley really stole the show with his micro robots. These robots ranged from a hexapod (think insect that is controlled by MEMS actuators and machine learning algorithms) to a quadcopter that used the Biefeld-Brown effect, a high-voltage ionic wind that is popular with UFO enthusiasts, to generate lift. Professor Pister’s talk also alluded to our xcore.ai poster which used the metric ‘micro Joules per inference.’
Day 2 started with a session on tinyML hardware and a presentation from KAIST, featuring a dystopian Robocop body-camera capable of automatically identifying criminals.
The following session from Samsung was extremely memorable, with the most impressive benchmark from across the Summit – 11.5 TOPS (trillions of operations per second) per watt from their new NPU (neural processing unit).
Following their press release earlier in the week, Arm presented their two new IP cores, a neural network accelerator (Ethos-U55) and a new processor core (Cortex-M55). Syntiant also delivered a presentation on their tiny, low power wake word chip, capable of performing always-on processing in the micro watts. The panel discussion on day 2 was not for the faint-of-heart and went in-depth on the range of bleeding edge memory technologies (some of which have been bleeding edge for decades) and in-memory compute. This certainly represents the future of truly tinyML with absolutely minimal power consumption. Of course, challenges arise with programmability, and the need to have deep analog design as part of the overall product (from “digital design” to programming models to noise-aware training).
Last but not least was a session on optimizing algorithms for tinyML. There was a lot of talk of compression (both hardware-enabled lossless compression of weights and dimensionality reduction through SVD techniques) and exploiting sparsity. We also got to see STM’s IDE for neural net deployment. This was an impressive package but took a slightly different approach to that favoured by XMOS and others (offline graph transformer and custom operators that are supported by TFLite Micro). The audience also expressed angst in the apparent fragmentation of programming tools/models from different hardware vendors but getting large fields to agree on common standards is a Sisyphean task which brings us to the penultimate talk given by MLPerf. MLPerf is a community that strive to define and publish fair and relevant benchmarks for machine learning platforms in training and inference that is now supporting tiny platforms like xcore.ai (they too acknowledged the difficulty in talking about power benchmarks).
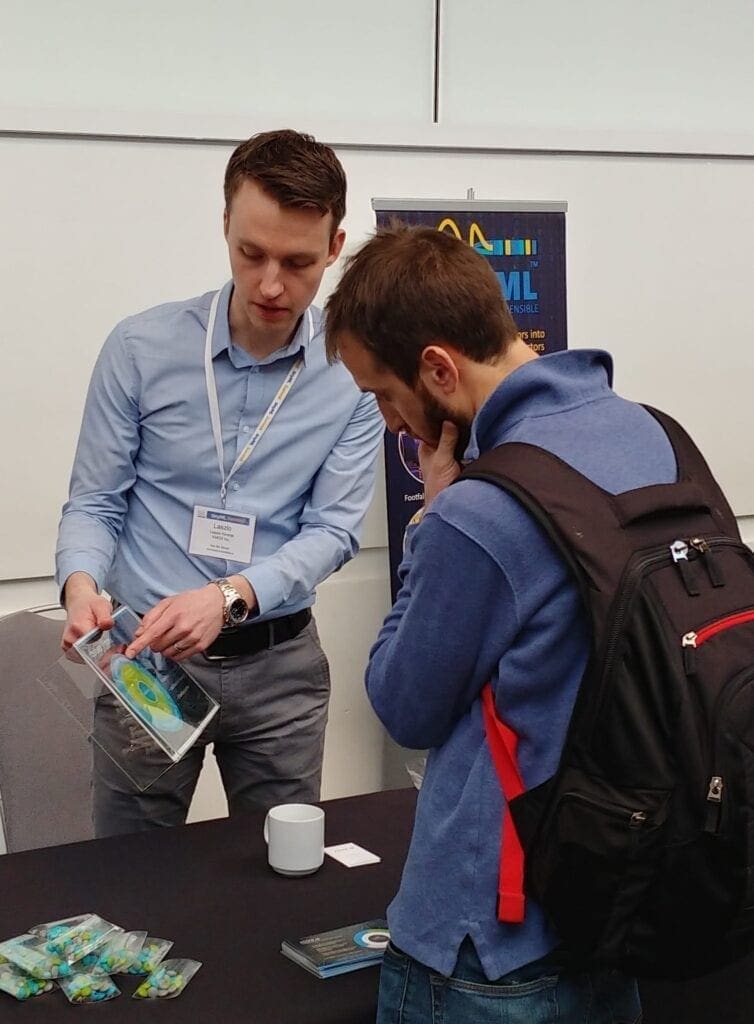
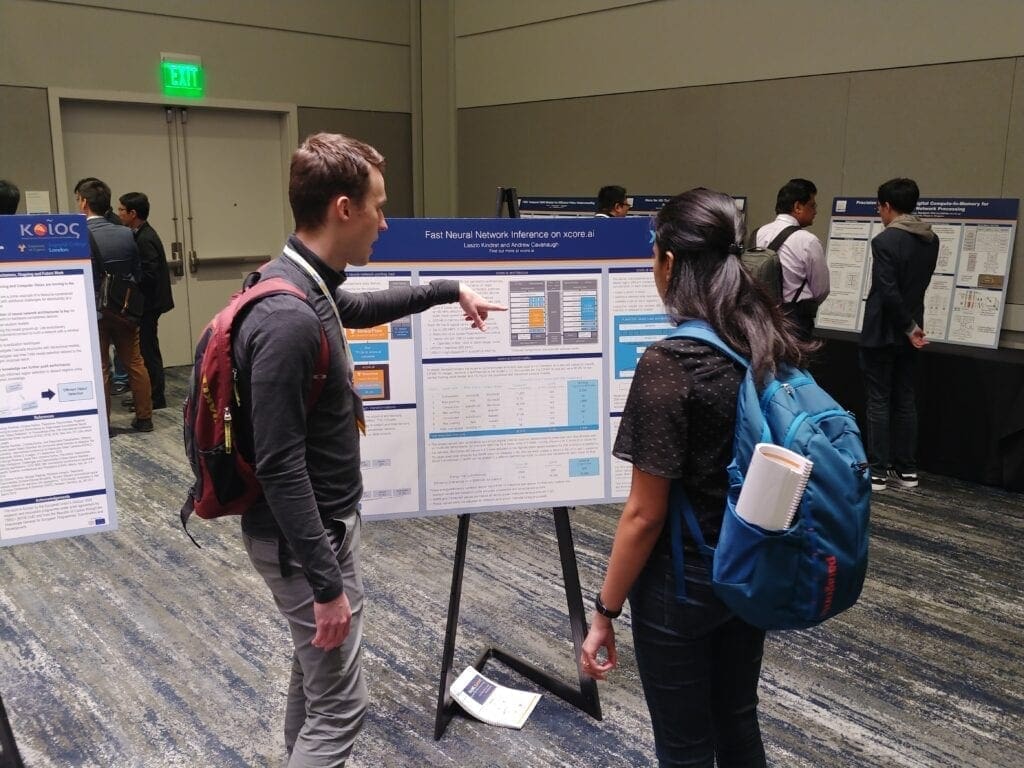
The interactions at our booth and poster, and during breaks were wonderful. Delegates represented a deeply technical community that is looking to solve a wide array of problems. While there were a ton of platforms being presented there were very few cases where more than one or two companies were vying for the same market segment in terms of power/performance. XMOS was also quickly recognised for our high flexibility, high performance, and low cost – with good energy efficiency.
Many thanks to the organisers of the tinyML Summit – we had a great few days, and look forward to 2021!